The journal Frontiers of Environmental Science & Engineering released an article on September 28th, 2023, which details how Georgia Institute of Technology researchers created a novel machine learning (ML) model to enhance Free Chlorine Residual (FCR) level forecasting.
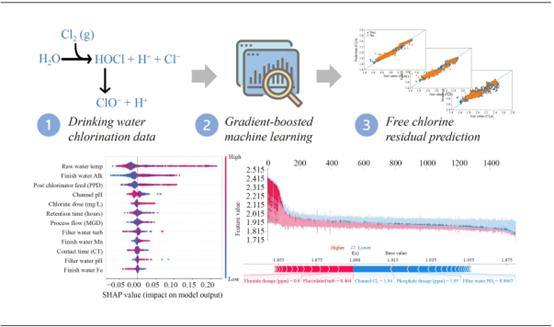
Graphic abstract. Image Credit: Frontiers of Environmental Science & Engineering
The model is trained on a year’s worth of data from a real, full-scale chlorine disinfection system in Georgia, USA, using a gradient-boosting machine learning technique called CatBoost. This data included operational process data as well as a range of water quality metrics.
The research team used a four-phase modeling strategy in this ground-breaking work, systematically moving through iterations incorporating rolling average models, parameter consolidation, and intuitive parameter models from a base case model. The models are carefully refined through the use of Bayesian optimization and cross-validation to improve the prediction performance.
The final model’s effectiveness is demonstrated by its remarkable coefficient of determination (R2) of 0.937. The utilization of the SHapley Additive explanation (SHAP) approach is crucial to the achievement of this goal, as it revealed the noteworthy, albeit counterintuitive, influence of normal drinking water treatment (DWT) operating parameters on the accuracy of Free Chlorine Residual (FCR) forecast.
Highlights
- A 98 MGD water treatment plant’s chlorination unit provided the annual statistics
- The prediction of free chlorine residuals was done using machine learning techniques
- With an R2 of 0.937, the final model iteration produced a good prediction value
- It was discovered that non-intuitive parameters had a considerable impact on predictions
The groundwork for data-driven supervision in DWT disinfection is established by this study. It offers fresh approaches to process monitoring that can provide plant supervisors with more insight into how to administer safe chlorine dosage. Furthermore, by adapting the methodology to other facets of water treatment and management, drinking water delivery systems around the globe could grow safer and more effective.
US Department of Agriculture’s National Institute of Food and Agriculture, Agriculture and Food Research Initiative, Water for Food Production Systems (2018-68011-28371); National Science Foundation (USA) (1936928, 2112533); US Department of Agriculture’s National Institute of Food and Agriculture (2020-67021-31526); US Environmental Protection Agency (840080010) funded the study.
Journal Reference
Helm, W., et al. (2023). Development of gradient boosting-assisted machine learning data-driven model for free chlorine residual prediction. Frontiers of Environmental Science & Engineering. doi.org/10.1007/s11783-024-1777-6
Source: https://www.gatech.edu/