The variable and stochastic character of wind energy distinguish it from other renewable resources. As a result, wind energy generation forecasting is critical for power system reliability and balancing supply and demand. This article will look at how machine learning has made wind energy more predictable and recent advancements in this field.
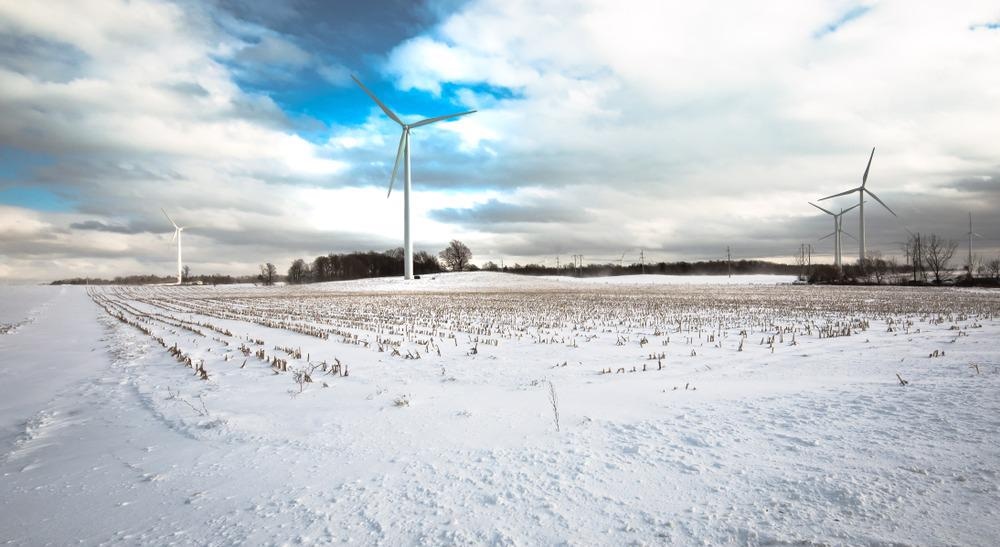
Image Credit: Chinnasut Nhurod/Shutterstock.com
Wind energy has gained a lot of attention because of its abundant resources and efficient power-producing technology.
However, large-scale strong and uncontrollable wind could undermine the stability of the power grid due to the uncertainty and randomness of the wind.
Wind energy generation is influenced by external and internal elements such as wind speed and direction, humidity, temperature, and pressure of the environment. Therefore, predicting wind generation enables the control, maintenance, management, and planning of electrical energy dispatch.
The Role of Machine Learning in Wind Energy Applications
Machine learning can assist wind farm operators in making better informed, data-driven decisions. As a result, there may be a better match between output and demand for electricity.
To deliver reliable prediction, machine learning analyzes current and historical weather data. Companies use this data to manage energy systems. They produce renewable energy and store it if the wind forecast is favorable. If it is poor, firms adjust their load accordingly.
Machine learning approaches have been quickly developed in recent years, and they have lately been employed for wind energy prediction. Machine learning performs better than traditional statistical prediction models in terms of feature extraction and model generalization.
Without being explicitly coded, machine learning systems can recognize structures and patterns in data sets, develop mathematical models to describe these relationships, and utilize them to make predictions or judgments.
Research and Development
Google and DeepMind Algorithm
To make wind power a more predictable energy source, Google and DeepMind used machine learning algorithms to 700 megawatts of wind generating capacity in the United States. Early data indicates that machine learning has increased the value of wind energy by approximately 20%.
Even though the algorithm is still being modified, Google believes that its usage of machine learning in wind farms has yielded positive results. The preliminary findings suggest that machine learning can improve the predictability and value of wind energy.
Machine learning also helps wind farm owners better assess how well their energy output will perform and satisfy the demand for electricity using more accurate data.
Vaisala Forecaster for Wind Energy
Vaisala, an environmental monitoring business, provides wind project evaluations, among other services.
In the wind energy industry, the Vaisala Forecaster for wind energy provides extraordinarily precise wind forecasts to successfully manage investments, decrease risk, and achieve a competitive advantage in the market.
Statistical techniques, highly specialized mesoscale Numerical Weather Prediction (NWP) models, machine learning models, and publically available predictions are used to create wind energy forecasts.
Numerical Weather Prediction (NWP) by Vaisala uses current weather conditions to model future weather and offer proxy data in locations where direct observations are not possible.
These models, which are based on complicated fluid dynamics and employ data from long-term wind measurements, anticipate wind energy generation by tapping into a variety of data sets.
MIT Machine Learning Model
Researchers at the Massachusetts Institute of Technology (MIT) have created a method using machine learning that can more quickly forecast changes in wind speed over a certain length of time. The method makes it easier for renewable energy start-ups to locate potential wind farms.
The MIT team estimated wind speeds three times more accurately for the following two years with only three months of historical data for a specific wind farm location than existing models could with eight months of data.
The researchers have since improved their model by experimenting with different methods of estimating joint distributions. Additional data from the Museum of Science suggests that their improved approach could increase the accuracy of their forecasts by as much as twofold.
Rutgers University Machine Learning Algorithm
Researchers at Rutgers University created a machine-learning algorithm to predict offshore wind power using physics-based simulators and real-world weather data. In addition, they established a sensitivity analysis methodology to find and anticipate the primary factors that contribute to environmental uncertainty in offshore wind in their study.
A machine learning model merges the output of a physics-based simulator with real-world meteorological data taken from a fleet of buoys installed in New Jersey to power this sensitivity analysis.
The buoys are located near at least three potential offshore wind projects and will contribute around 2.8 gigawatts of offshore wind power to the United States by 2024. The team's research indicated that waves are essential in predicting wind direction and speed.
Future Outlooks of Machine Learning in Wind Applications
Wind energy has become an important source of carbon-free electrical energy in numerous countries over the last decade. However, this energy remains an unreliable source because of the wind's unpredictability and inconsistent behavior.
An artificial intelligence model that can accurately forecast the unpredictability of wind energy production will aid in managing supply and demand changes. Using machine learning, wind power may become more profitable, and carbon-free energy could be more widely used in power systems worldwide.
References and Further Reading
Chen, X., Zhang, X., Dong, M., Huang, L., Guo, Y., & He, S. (2021). Deep Learning-Based Prediction of Wind Power for Multi-turbines in a Wind Farm. Frontiers in Energy Research, 403. https://doi.org/10.3389/fenrg.2021.723775
Elyasichamazkoti, F., & Khajehpoor, A. (2021). Application of machine learning for wind energy from design to energy-Water nexus: A Survey. Energy Nexus, 2, 100011. https://doi.org/10.1016/j.nexus.2021.100011
Golparvar, B., Papadopoulos, P., Ezzat, A. A., & Wang, R. Q. (2021). A surrogate-model-based approach for estimating the first and second-order moments of offshore wind power. Applied Energy, 299, 117286. https://doi.org/10.1016/j.apenergy.2021.117286
Hardesty, L. (2015). Siting Wind Farms More Quickly, Cheaply. [Online]. MIT News Office. Available at: https://news.mit.edu/2015/siting-wind-farms-quickly-cheaply-0717 (Accessed on 18 April 2022).
Khatib, T., Deria, R., & Isead, A. (2020). Assessment of Three Learning Machines for Long-Term Prediction of Wind Energy in Palestine. Mathematical Problems in Engineering, 2020. https://doi.org/10.1155/2020/8303152
Vaisala. (2020). Forecaster For Solar and Wind Energy. [Online]. Available at: https://www.vaisala.com/en.
Disclaimer: The views expressed here are those of the author expressed in their private capacity and do not necessarily represent the views of AZoM.com Limited T/A AZoNetwork the owner and operator of this website. This disclaimer forms part of the Terms and conditions of use of this website.