Nuclear energy is gaining interest as a renewable alternative to solar and wind power. However, ensuring its safety is crucial for widespread adoption and combating climate change. With the rapid advancement of artificial intelligence (AI) technology, opportunities and challenges arise for enhancing nuclear reactors' safety and economic aspects. This article explores the potential of utilizing AI to make nuclear energy safer and more efficient.
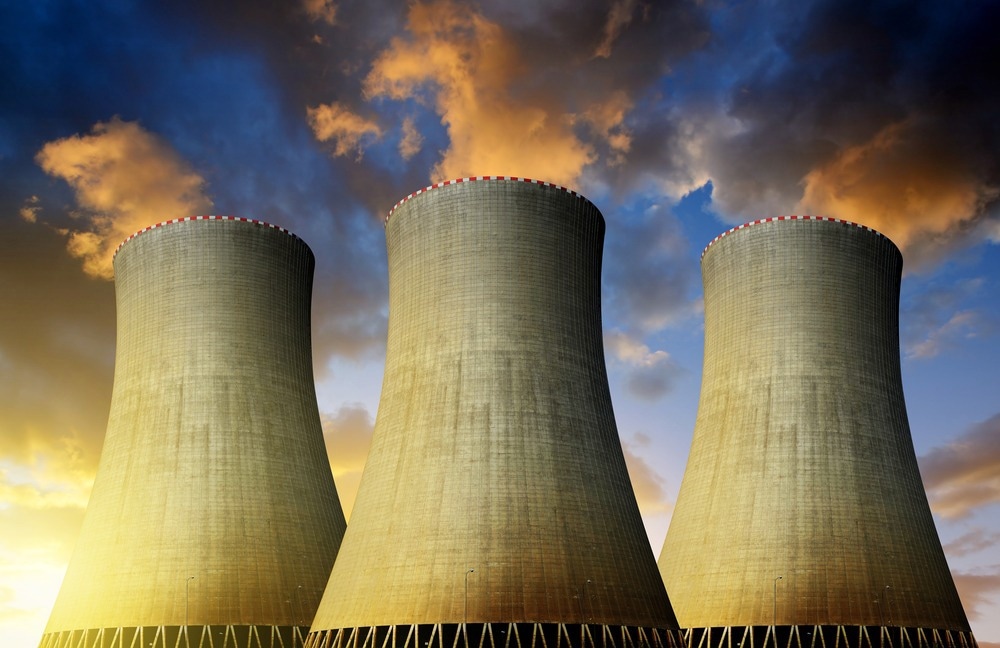
Image Credit: jaroslava V/Shutterstock.com
Governments worldwide are promoting low-carbon policies in alignment with the goals of the Paris Agreement, aimed at reducing greenhouse gas emissions and fostering sustainable development.
While wind and solar sources are renewable, their intermittency and dependence on energy storage technologies present challenges. As a result, nuclear energy has emerged as a reliable alternative to solar and wind energy for providing limitless clean energy.
However, challenges and recent incidents, such as Three Mile Island and Chernobyl, have highlighted the importance of ensuring safety in nuclear power plants (NPPs). Human errors, high workloads, and information overload have been significant concerns, leading to failures and system downtime.
With the advancement of data analysis tools and computing capabilities, the nuclear power industry has embraced AI, deep learning, and machine learning techniques to enhance safety, optimize plant operation and maintenance, and develop advanced technologies.
AI methodologies enable physics-based predictive analysis, optimizing design, manufacturing, construction, operation, and fault detection. By analyzing data from various sources, such as sensors and drones, a digital twin can be created to support automation, inspection, risk monitoring, reactor design, and predictive maintenance. In addition, augmented and virtual reality technologies contribute to training and reducing human-related errors during operation and maintenance.
How is Artificial Intelligence Making Nuclear Energy Safer?
Predictive maintenance
Nuclear power plants traditionally rely on regular preventive maintenance, which can lead to excessive maintenance and increased costs. Researchers have innovated an AI-based Prognostics and Health Management (PHM) framework to improve safety and cost-effectiveness.
This framework predicts equipment's residual useful life (RUL), enabling a shift from fixed to on-demand maintenance. In addition, this framework is employed as the direct mapping between sensor data and RUL, or degenerate trajectory modeling using machine learning algorithms.
These methods optimize maintenance strategies, reduce resource wastage, and minimize risks associated with excessive maintenance.
Decision support systems
Smart support systems have been extensively researched to enhance operator decision-making in nuclear power plants. These systems aim to alleviate information overload and stress on operators during abnormal situations.
Fault diagnosis systems, including artificial neural networks (ANNs) and knowledge-based systems, help quickly identify faults and offer accident-related information. Deep learning models have shown effectiveness in accurately predicting accidents, while ANNs combined with knowledge-based modules improve fault diagnosis accuracy.
Declarative AI approaches and uncertainty estimation techniques contribute to reliable decision-making. In addition, these systems reduce human error and operator stress, ensuring safe and efficient plant operation.
Fuel management
AI has significantly improved fuel management in the nuclear industry by optimizing fuel assembly design, core reload patterns, and fuel performance assessment. In addition, it enables a comprehensive analysis of fuel properties and their interdependencies, ensuring safe and efficient operation.
AI-based ant-colony models, genetic algorithms, and particle swarm optimization enable generating multiple viable solutions that closely align with reference parameters. This empowers power plant operators with a wider selection of choices for optimizing fuel bundle burnups and determining discharge burnups.
Operator monitoring system
AI advancements have revolutionized operator monitoring systems in nuclear power plants, significantly reducing human error and enhancing safety. Traditional approaches to assessing operators' fitness for duty have been subjective and infrequent. However, AI-based systems, such as bio-monitoring systems, facial expression analysis, computer vision technologies, and wearable device sensors, enable continuous monitoring of operators' physical and mental performance.
These technologies enable immediate performance estimation, predict and minimize human error risks, monitor cognitive factors, simplify measurement processes, detect anomalous behavior, and improve situational awareness and team coordination.
Sensor fault detection systems
Reliable sensor data is crucial for accurate decision-making in nuclear power plants to ensure safety. Sensor failures or incorrect readings can lead to potential hazards and erroneous actions.
AI has improved sensor fault detection systems in nuclear power plants by using probabilistic neural networks, fuzzy logic, and machine learning models to identify and diagnose instrument failures. These systems monitor deviations between predicted values and observed plant parameters, enabling the detection and isolation of sensor faults.
Choi and Lee designed a sensor fault-tolerant accident diagnosis system using a recurrent neural network with decay and an iterative random forest model for robust fault identification and data recovery in nuclear power plants during abnormal conditions and shutdown or startup operations.
Automated safety assessment and text analysis systems
AI has improved the nuclear power plant's operation and safety by enhancing safety evaluation methodologies and probabilistic risk assessments (PRAs) and automating the analysis of event reports using NLP techniques.
It has enabled the identification of errors, violations, and procedure issues, improved team processes, and incorporated organizational factors into risk assessments, improving safety analysis efficiency.
Researchers have developed new approaches, such as adopting early warning scores from the medical field and using Bayesian networks to handle uncertainties and improve the accuracy of safety evaluations.
AI techniques, including deep learning, have obtained plausible accident scenarios and reduced uncertainty in PRAs, leading to more comprehensive and dynamic safety analyses.
Recent Research and Implementation of AI for Enhancing Nuclear Energy Safety
AI-enabled anomaly detection for human errors in nuclear power plants
A recent study published in Nuclear Engineering and Technology aimed to detect human errors in nuclear power plants using a generative adversarial network (GAN)-based unsupervised anomaly detection model.
Researchers analyzed sensor and vibration data from a nuclear power plant and correlated them to identify anomalies potentially caused by human error.
They evaluated the model's performance under different assumed anomaly rates and validated their approach using an external dataset from a testbed, intentionally introducing erroneous data associated with a human error during the collection process.
The proposed GAN-based model outperformed the state-of-the-art models, such as isolation forest and one-class support vector machine, in detecting anomalous data.
The proposed framework can enhance human error mitigation by alerting the operators of potential errors, promoting further evaluation or retaking of measurements, and laying the groundwork for future studies on human error identification for safe nuclear plant operations.
AI-based crack detection system in nuclear reactors
Purdue University’s engineers developed an AI system to enhance nuclear reactor safety. Their study, published in IEEE Transactions on Industrial Electronics, introduced a deep learning framework, Naïve Bayes-convolutional neural network, to identify cracks in reactor surfaces by analyzing overlapping patches of the individual video frames.
The AI system tracks each crack across consecutive frames using a data fusion algorithm, resulting in robust decision-making. As a result, it achieved a remarkable 98.3% success rate in crack identification, surpassing current identification techniques.
This study significantly improves safety inspections of nuclear power plant components by automating crack detection through AI. It enhances safety and efficiency and reduces reliance on human technicians, addressing the limitations of current manual inspection methods.
Using artificial intelligence to predict and avoid disruptions in fusion facilities
In a study published in Physics of Plasmas, an international team of scientists led by a graduate student at the Princeton Plasma Physics Laboratory successfully predicted and prevented disruptions in fusion reactions using AI.
By training an AI machine learning algorithm on previous experiments conducted at the DIII-D National Fusion Facility, the researchers forecasted the likelihood of disruptions in real-time and prevented them.
This achievement is significant as disruptions can halt fusion reactions and cause severe damage to fusion facilities. In addition, the study contributes to the development of disruption prediction and avoidance methods for fusion devices like ITER, aiding in the practical implementation of fusion energy.
Future of Artificial Intelligence in the Nuclear Industry
The practical implementation of AI in nuclear power plants is still a work in progress due to the longstanding use of computational intelligence in the industry, hindered by the lack of reliable databases for critical scenarios and human-automation interaction.
AI has significant potential for technological advancement in the nuclear sector through cross-sector collaboration, standardization, and international cooperation. These efforts will facilitate realizing AI's full potential in improving nuclear energy safety, security, and efficiency.
References and Further Reading
Chen, F. C., & Jahanshahi, M. R. (2017). NB-CNN: Deep learning-based crack detection using convolutional neural network and Naïve Bayes data fusion. IEEE Transactions on Industrial Electronics. https://doi.org/10.1109/TIE.2017.2764844
Fu, Y., Eldon, D., Erickson, K., Kleijwegt, K., Lupin-Jimenez, L., Boyer, M. D., ... & Kolemen, E. (2020). Machine learning control for disruption and tearing mode avoidance. Physics of Plasmas. https://doi.org/10.1063/1.5125581
Gursel, E., Reddy, B., Khojandi, A., Madadi, M., Coble, J. B., Agarwal, V., ... & Boring, R. L. (2023). Using artificial intelligence to detect human errors in nuclear power plants: A case in operation and maintenance. Nuclear Engineering and Technology. https://doi.org/10.1016/j.net.2022.10.032
Huang, Q., Peng, S., Deng, J., Zeng, H., Zhang, Z., Liu, Y., & Yuan, P. (2023). A review of the application of artificial intelligence to nuclear reactors: Where we are and what's next. Heliyon. https://doi.org/10.1016/j.heliyon.2023.e13883
Sethu, M., Kotla, B., Russell, D., Madadi, M., Titu, N. A., Coble, J. B., ... & Khojandi, A. (2023). Application of Artificial Intelligence in Detection and Mitigation of Human Factor Errors in Nuclear Power Plants: A Review. Nuclear Technology. https://doi.org/10.1080/00295450.2022.2067461
Suman, S. (2021). Artificial intelligence in nuclear industry: Chimera or solution? Journal of Cleaner Production. https://doi.org/10.1016/j.jclepro.2020.124022
Choi, J., & Lee, S. J. (2020). Strategy for the accident diagnosis in sensor error states. Trans. Korean Nuclear Soc, 20, 20. https://www.kns.org/files/pre_paper/43/20S-565-%EC%B5%9C%EC%A0%95%ED%9B%88.pdf
Disclaimer: The views expressed here are those of the author expressed in their private capacity and do not necessarily represent the views of AZoM.com Limited T/A AZoNetwork the owner and operator of this website. This disclaimer forms part of the Terms and conditions of use of this website.