Wind-generated electricity is poised to assume a pivotal role in the global energy landscape as the world shifts away from fossil fuels towards renewable sources. Wind speed stands as a crucial parameter for assessing the wind energy potential in a given location. However, accurately predicting wind speed remains a formidable challenge.
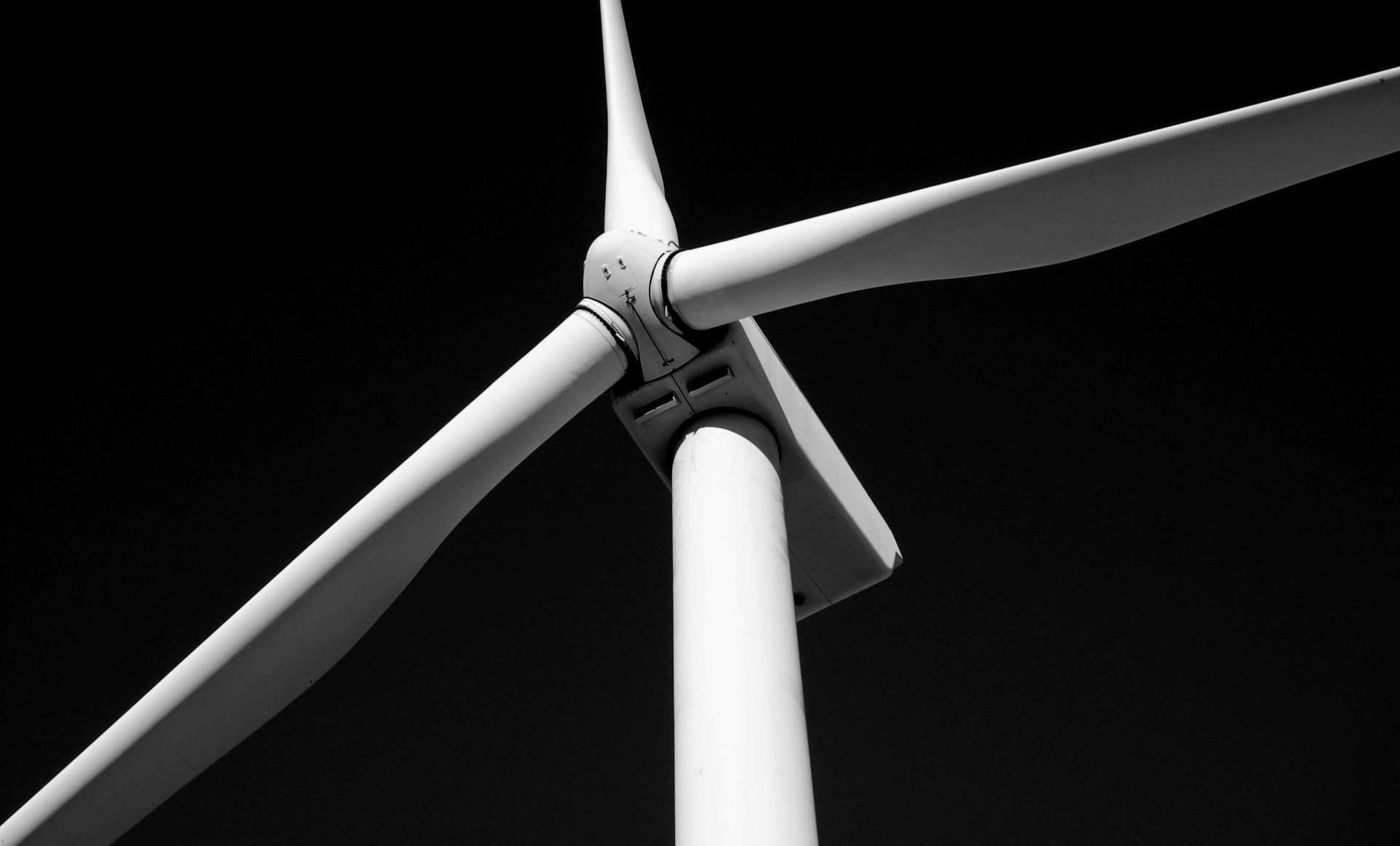
Image Credit: Matt Artz, Unsplash
This poses a significant obstacle for utilities that depend on dependable estimates to efficiently power their grids.
Numerous models currently exist for forecasting wind speed, each offering varying degrees of accuracy and reliability. In a study led by Concordia University and published in the journal Energies, researchers from the Department of Building, Civil, and Environmental Engineering at the Gina Cody School of Engineering and Computer Science introduce a hybrid method that combines multiple types of models to address this challenge.
The novel approach employs data analysis and information derived from two distinct sources: a Weibull probability distribution and a numerical weather prediction (NWP). The Weibull distribution, in this context, estimates wind speed probabilities by considering historical data and other relevant variables. In contrast, the NWP relies on physical principles and employs a complex algorithm to forecast future behavior.
NWPs are commonly employed by weather services and applications to provide hourly and daily weather forecasts.
However, despite their strengths, Shirzadi points out that these models come with noteworthy limitations when attempting to adapt them for power generation purposes.
The stochastic behavior of renewables and their fluctuations make designing and operating a microgrid very challenging. There is no special pattern or seasonality that you can capture easily. We need a very strong model in order to predict wind power generation.
Navid Shirzadi, Research Scientist, Department of Building, Civil and Environmental Engineering, Concordia University Montreal
The scientists initially fused the Weibull probabilities into a Long Short-Term Memory (LTSM) model. An LTSM is a kind of persistent neural network useful for time-series analysis. To strengthen their model, the scientists then added data from the NWP.
The initial fusion of LSTM and the Weibull distribution produced encouraging outcomes, according to Shirzadi. These results were further bolstered by the incorporation of the NWP. When compared to non-hybridized LSTM predictions over a 48-hour timeframe, the integration of NWP led to a significant 32% reduction in forecasting errors.
Gusts for Growth
Despite the global expansion of wind power, there is still a substantial amount of work to be done to reach the international goal of achieving net-zero emissions by 2050. The International Energy Agency has highlighted that to attain this net-zero objective by mid-century, it is necessary to produce 7,400 terawatt-hours (TWh) of electricity from wind power alone by the end of this decade. Currently, global wind electricity generation amounts to 2,100 TWh.
This underscores the need for consistent, robust, and sustained investment in wind power for the foreseeable future to bridge this considerable gap.
Associate Professor and Co-Author Fuzhan Nasiri expresses his belief that this research, in addition to other studies generated by members of Concordia University's Next Generation Cities Institute and Volt-age initiative, has the potential to make a meaningful contribution to Concordia's decarbonization endeavors.
As Concordia progresses towards its own Net Zero Emissions by 2050 commitments, it is positioning itself as a prominent leader in Canada's efforts to address climate change and reduce carbon emissions.
We must diversify our energy sources. Local capacities are a part of that. We cannot rely solely on the existing grid, which can be vulnerable to natural disasters like ice storms. It adds a buffer during power outages. So, it is extremely important to have some precise idea about wind speed to see which area has the highest potential for a turbine’s operational efficiency.
Fuzhan Nasiri, Associate Professor & Associate Chair, Building, Civil, and Environmental Engineering, Concordia University Montreal
“Energy management increasingly gains in importance, as intermittent renewables need to match the demand all the time. Accurate wind speed prediction is the basis to best predict the variable power generation and adapt the loads and storage usage,” noted Ursula Eicker, Canada Excellence Research Chair in Smart, Sustainable and Resilient Cities and Communities, and Shirzadi’s main supervisor.
Concordia’s CERC team postdoctoral researchers Ramanunni Menon and Pilar Monsalvete, and Anton Kaifel at the Center for Solar Energy and Hydrogen Research in Stuttgart, Germany, also took part in the research.
This study received funding from the NSERC Discovery grant program and the Tri-Agency Institutional Program Secretariat.
Journal Reference
Shirzadi, N., et al. (2023). Smart Urban Wind Power Forecasting: Integrating Weibull Distribution, Recurrent Neural Networks, and Numerical Weather Prediction. Energies. doi.org/10.3390/en16176208