In a recent study published in the journal Scientific Reports, researchers presented a comprehensive analysis of various hybrid renewable energy system (HRES) configurations for powering rural communities in Ethiopia. They employed state-of-the-art optimization techniques to determine the optimal sizing and integration of solar photovoltaic (PV), wind, and pumped hydro-energy storage (PHES) systems to deliver a cost-effective and reliable electricity supply to this off-grid region.
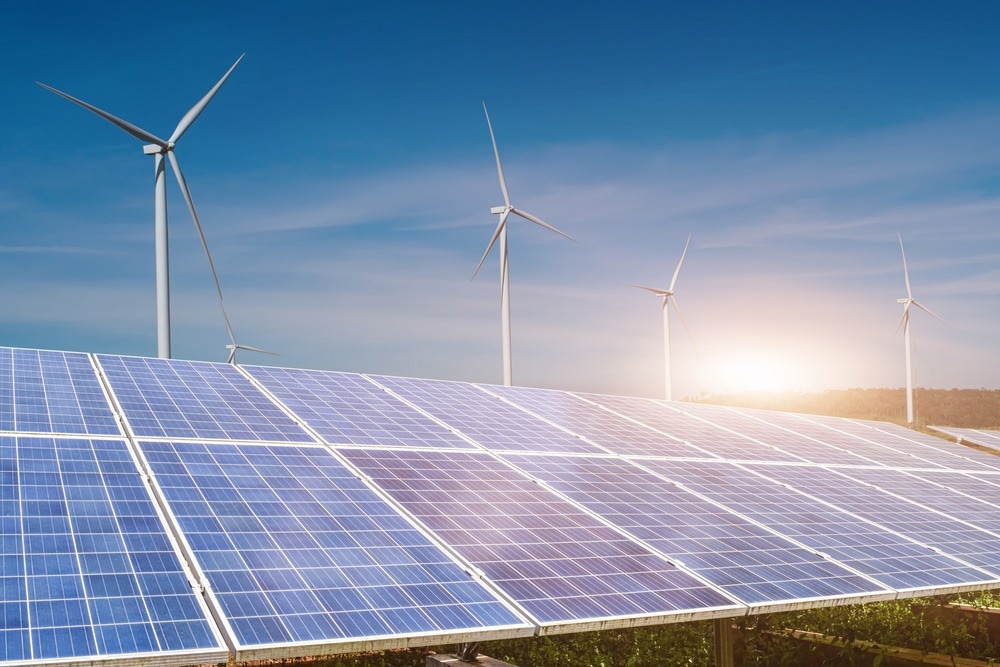
Image Credit: Porstocker/Shutterstock.com
Hybrid Renewable Energy Systems: A Promising Solution
HRES have emerged as a promising solution to address the energy challenges faced by remote and underserved communities, particularly in developing nations.
By integrating multiple renewable energy sources and storage technologies, HRES can harness the complementary nature of these resources to provide a reliable and sustainable power supply.
Incorporating PHES, in particular, can enhance the system's flexibility and resilience, mitigating the intermittency inherent in solar and wind power generation.
Minimizing Total Life Cycle Cost and Cost of Energy
The study authors developed an efficient and reliable HRES solution for Gaita Selassie, a rural village in the Dangila district of Ethiopia. Their primary focus was to minimize the total life cycle cost (TLCC) and the cost of energy (COE) while ensuring a dependable power supply, as indicated by the loss of power supply probability (LPSP).
Employing a comprehensive approach encompassing technical and economic factors, the study utilized advanced optimization techniques to determine the optimal configuration of renewable energy sources and storage systems. Specifically, they employed two advanced optimization algorithms: the Multiobjective Gray Wolf optimization (MOGWO) and the Multiobjective Grasshopper optimization algorithm (MOGOA).
These techniques were used to optimize the sizing of PV, wind, and PHES components within the HRES. By leveraging these algorithms, the researchers aimed to find the most suitable configuration that balances technical efficiency and economic feasibility while meeting the energy demands.
The paper evaluated three HRES scenarios: PV-PHES, Wind-PHES, and PV-Wind-PHES. Each scenario combines various renewable energy sources with PHES. For example, PV-PHES integrates PV panels and PHES, Wind-PHES combines wind turbines with PHES, and PV-Wind-PHES includes PV panels, wind turbines, and PHES.
The authors analyzed each scenario's technical and economic feasibility, considering factors such as solar irradiation, wind speed, load demand, and component cost.
The optimization algorithms were used to determine the optimal size of each component, including the number of PV panels, wind turbines, and the capacity of the PHES system.
Research Findings
The paper showcased the superior performance of the MOGWO algorithm in optimizing the HRES configurations. In the PV-PHES scenario, the MOGWO algorithm determined an optimal system with 13,889 solar panels, a PHES capacity of 1.84 MW, and an upper reservoir (UR) volume of 52,529 m³.
Moreover, the Wind-PHES scenario yielded an optimal configuration of 15 wind turbines and a PHES capacity of 1.65 MW with a UR volume of 45,800 m³. For the PV-Wind-PHES scenario, the MOGWO algorithm recommended 2,141 solar panels, five wind turbines, and a PHES capacity of 1.52 MW with a UR volume of 40,502.1 m³.
Comparative analysis of the optimization techniques revealed that the MOGWO algorithm outperformed the MOGOA algorithm in minimizing the COE and TLCC while maintaining a zero LPSP.
The PV-Wind-PHES scenario emerged as the most cost-effective solution, with a TLCC of €6,897,300 and a COE of €0.1261/kWh, representing a 38% and 18% reduction, respectively, compared to the Wind-PHES and PV-PHES scenarios.
The authors demonstrated that the PHES system was crucial in ensuring a reliable energy supply, particularly during low wind speed and solar irradiation periods. The PHES system effectively stored excess energy generated during peak periods and released it during periods of high demand, ensuring a consistent and reliable electricity supply.
Electrifying Rural Communities
The study outcomes significantly affect electrifying rural communities in Ethiopia and similar off-grid regions. The optimized HRES configurations can offer a reliable and cost-effective solution to address the pressing energy needs of these underserved areas. By harnessing the abundant solar, wind, and hydropower resources available in the region, the proposed system can deliver a sustainable and uninterrupted power supply, tackling the challenges of limited grid access and reliance on traditional energy sources.
Moreover, integrating PHES technology within the HRES enhances the system's reliability and flexibility. This ensures that energy demand is met even during fluctuating renewable energy generation periods. This approach makes the system more resilient and adaptable to changing environmental conditions by effectively mitigating the intermittency inherent in solar and wind power.
Conclusion
The researchers highlighted various HRES configurations for rural electrification, with PV-Wind-PHES emerging as the most effective solution. Its successful implementation could set a standard for sustainable energy initiatives globally.
The study suggested integrating demand-side management strategies and advanced energy storage technologies to further enhance system efficiency and resilience. They proposed incorporating environmental impact assessments and intelligent grid technologies to improve the system's sustainability and adaptability.
Journal Reference
Agajie, E.F., Agajie, T.F., Amoussou, I. et al. Optimization of off-grid hybrid renewable energy systems for cost-effective and reliable power supply in Gaita Selassie Ethiopia. Sci Rep 14, 10929 (2024). https://doi.org/10.1038/s41598-024-61783-z
Disclaimer: The views expressed here are those of the author expressed in their private capacity and do not necessarily represent the views of AZoM.com Limited T/A AZoNetwork the owner and operator of this website. This disclaimer forms part of the Terms and conditions of use of this website.