A recent study in the journal Energies explored how deep learning (DL) techniques can forecast electricity production from renewable sources like solar and wind power. The researchers aimed to develop an efficient and accurate prediction model for energy output, which is crucial for effective energy management and planning amid the growing demand for sustainable solutions.
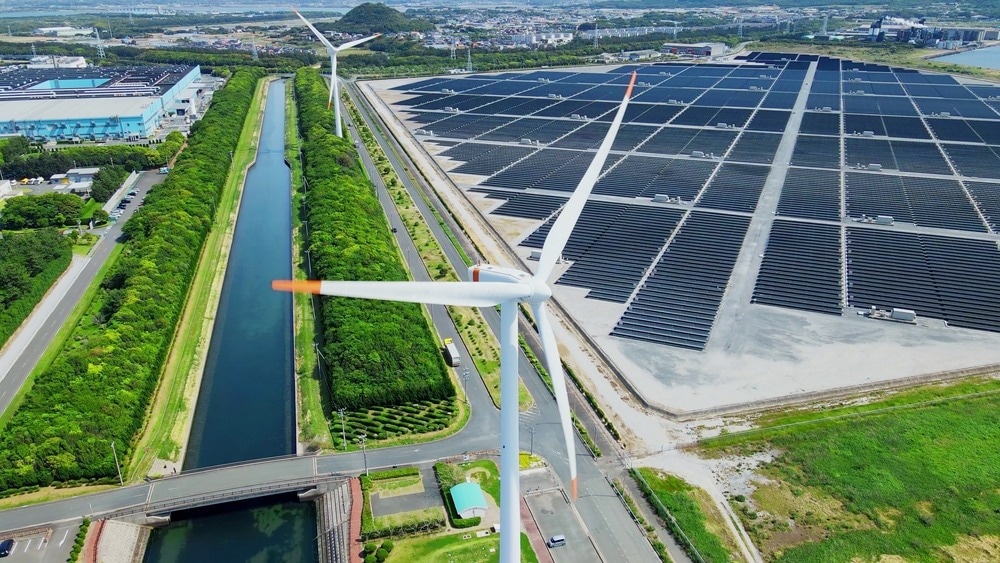
Image Credit: metamorworks/Shutterstock.com
Background
Renewable energy sources such as solar, wind, biomass, geothermal, hydroelectricity, and wave energy are becoming increasingly important alternatives to fossil fuels. Fossil fuels, although still dominant in electricity generation, significantly pollute the environment and harm animal, human, and plant health. Transitioning to renewable energy is essential to reduce these negative impacts and achieve sustainable production. However, the intermittent nature of renewable sources, particularly solar and wind power, challenges grid stability and reliable energy supply. Therefore, accurate energy production forecasting is crucial for effective grid management and system integration.
Traditional forecasting methods, like the seasonal autoregressive integrated moving average (SARIMA) model, have been useful but often struggle to capture the complex dynamics of renewable energy production. This has led researchers to investigate machine learning (ML), especially DL, to enhance forecasting accuracy and reliability.
About the Research
In this paper, the authors used DL models to address the challenge of forecasting electricity production from solar and wind power plants. They compared two widely used models: long short-term memory (LSTM) and SARIMA. The goal was to predict energy production from three solar power plants and wind speed at two locations. The LSTM model, a type of recurrent neural network, is designed for sequential data and is well-suited for time series forecasting. In contrast, the SARIMA model is a statistical approach that captures seasonal and autoregressive patterns in data.
The researchers used three years of daily production data from three 1 MW solar power plants for solar energy forecasting. They used two years of data from two meteorological stations for wind speed forecasting. The data was divided into training and testing sets, with 70% used for training and 30% for testing. Furthermore, the performance of the models was assessed using various error metrics, including root mean square error (RMSE), coefficient of determination (R2)mean square error (MSE), mean absolute error (MAE), normalized mean square error (NMSE), and mean absolute percentage error (MAPE). The study also explored other DL architectures, such as convolutional neural networks (CNN), gated recurrent units (GRU), and hybrid models for wind speed forecasting.
Research Findings
The outcomes showed that the LSTM model was more accurate than the SARIMA model for predicting solar energy production. The LSTM model demonstrated higher accuracy, with MAPE values ranging from 59% to 81% across the three power plants, while the SARIMA model had MAPE values between 41% and 66%. This indicates that LSTM networks better capture the complex time dependencies in solar energy data.
For wind speed forecasting, the CNN-GRU hybrid model outperformed other DL models. It achieved high accuracy in weekly, monthly, and annual forecasts, with a MAPE of 5.48%, an R2 value of 0.9981, and an RMSE of 0.0127 for monthly forecasts. This suggests that combining CNNs and GRUs effectively captures spatial and temporal patterns in wind speed data, improving forecasting accuracy.
Applications
Accurate forecasting of renewable energy production is crucial for effective energy management and planning. This research can improve the efficiency of solar and wind power plants by enhancing planning and maintenance strategies. Accurate predictions can help operators optimize operations, reduce downtime and maintenance costs, and ensure a reliable energy supply.
Additionally, the study's findings can help develop advanced grid management systems, facilitating the integration of renewable sources into existing infrastructure. Better forecasting supports a smoother transition from fossil fuels to cleaner energy sources, contributing to a more stable and environmentally friendly energy system. By predicting energy production, grid operators can better manage supply and demand, optimize energy storage and dispatch, and support a cleaner energy future.
Conclusion
In summary, DL models effectively forecast electricity production from renewable sources. The LSTM model was particularly effective for solar energy forecasting, while the CNN-GRU hybrid model excelled at predicting wind speed. These results highlighted the importance of advanced ML techniques in enhancing the reliability and efficiency of renewable energy systems.
Future research should enhance these models' accuracy and reliability, explore new deep-learning architectures, incorporate more data sources, and refine data preprocessing methods. Additionally, real-time forecasting applications should be considered to assist grid operators in making better decisions based on changing weather conditions and energy demand.
Disclaimer: The views expressed here are those of the author expressed in their private capacity and do not necessarily represent the views of AZoM.com Limited T/A AZoNetwork the owner and operator of this website. This disclaimer forms part of the Terms and conditions of use of this website.
Source:
Karakan, A. Predicting Energy Production in Renewable Energy Power Plants Using Deep Learning. Energies 2024, 17, 4031. DOI: 10.3390/en17164031, https://www.mdpi.com/1996-1073/17/16/4031